Hear from our Chief Scientist and the Head of Data, Sheikh Fakhar Khalid and how the geospatial industry is on the brink of a transformative shift, driven by advancements in artificial intelligence and machine learning. Among these innovations, embeddings stand out as a game-changer, offering a revolutionary way to represent, integrate, and analyse diverse geospatial datasets. This blog explores how embeddings work, why they matter, and the unprecedented opportunities they unlock for geospatial workflows.
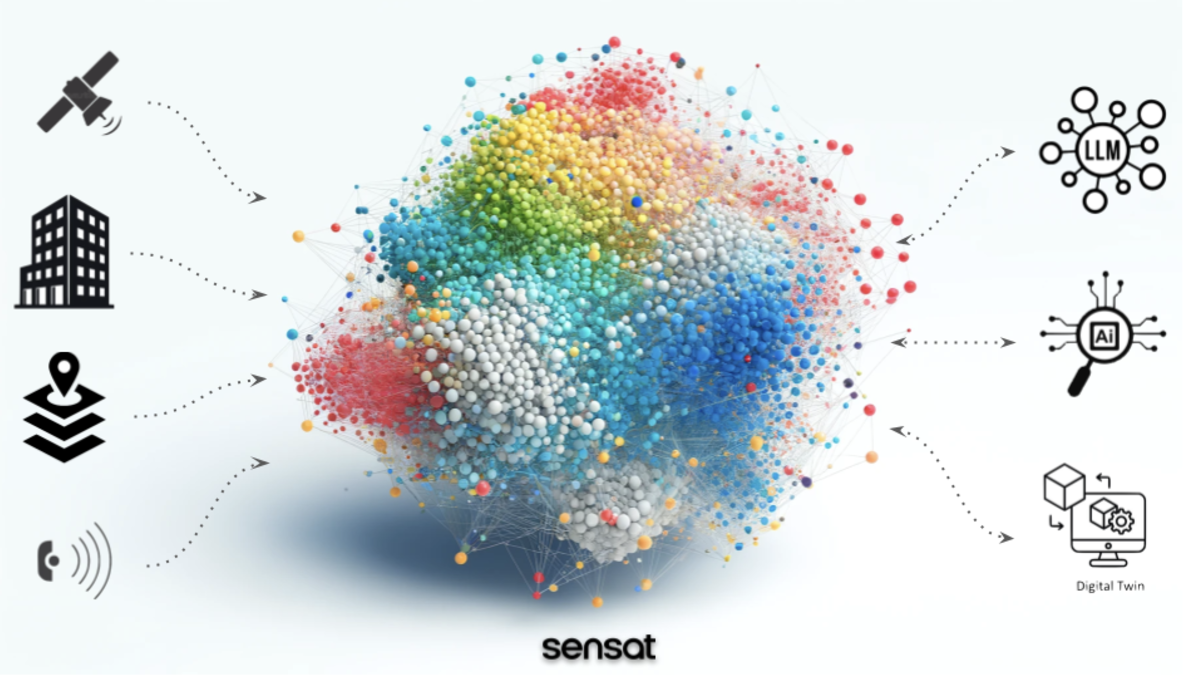
How Embeddings Will Revolutionise the Geospatial Data Industry
What if you could query the world as easily as you search the internet? What if finding the perfect location for a renewable energy project, analysing urban traffic patterns, detecting environmental changes or even designing an upgrade to an energy grid took minutes instead of weeks or months? This vision is no longer a distant dream but an imminent reality, thanks to the transformative power of vector embeddings - a technology poised to transform geospatial intelligence.
Traditionally, geospatial data structures have relied on explicit, rigid formats, coordinate pairs, topological graphs, and raster grids. These structures have served us well for decades, but we need a paradigm shift as the demand for more innovative, faster, and dynamic geospatial insights grows. The traditional formats are inherently limited by their static nature, reliance on specific tools, and the complexity of integrating diverse data types. Embeddings provide that shift, offering the promise of a geospatial data revolution.
What Are Embeddings?
At its core, an embedding is a mathematical way of representing data in a continuous, multi-dimensional vector space. In simpler terms, it transforms complex geospatial, geometric, and contextual information into a streamlined format that computers can understand. This format works like a smart map, organising data so that related pieces are grouped together. This helps computers understand the meaning and connections within the data. Embeddings act like a compact and intelligent version of this map, making it easier and super fast to analyse while keeping the full context and relationships intact.
For example, in designing an energy grid upgrade, embeddings integrate diverse data like GIS layers, satellite imagery, CAD designs, and textual reports. Embeddings go beyond mapping physical infrastructure locations and can also encode dynamic factors such as energy flow, demand variability, and environmental constraints into a machine-optimised format. This unified space allows planners to dynamically cross-reference multiple factors, enabling smarter decisions about energy routing, repair scheduling, and optimisation for carbon-neutral goals.
This shift from rigid, domain-specific data formats to a unified representation transforms how data is stored, queried, and analysed, paving the way for smarter, more integrated workflows.
How a Unified Embedding Space Works
A unified embedding space acts as a shared framework that transforms diverse data types such as imagery, text, GIS layers, and sensor readings into mathematical representations. Within this multidimensional space, similar entities, regardless of their origin or format, are positioned closer together. For instance, a satellite image depicting a dense forest, a textual description of ‘dense vegetation’ and a GIS polygon representing tree coverage are all encoded into the same vector space so that their relationships and similarities are preserved.
Advanced AI models create these embeddings by learning patterns and connections within the data. Unlike traditional methods where humans manually define categories or relationships, these models automatically uncover deeper insights that are hard to capture manually. For instance, instead of just identifying a forest, embeddings can also describe its ecological features, how it’s used, and how it changes over time, all without manual input.
This approach allows embeddings to capture semantic, contextual, and relational parameters, making them far richer and more flexible than traditional representations. By distilling complex, multi-source data into compact, machine-optimised structures, embeddings enable powerful cross-domain integrations and deeper insights, redefining the potential of geospatial data analytics.
Why Are Embeddings Transformative for Geospatial Data?
Embeddings are not just a technical upgrade, they represent a fundamental departure from traditional data structures in geospatial analysis, intelligence and data management. Embeddings enable interoperability by unifying diverse data types, such as satellite imagery, GIS layers, textual descriptions, and CAD models, into a single representation that preserves the unique characteristics, underlying patterns and the relationships between data. This seamless integration removes the need for complex conversions and allows for richer and more meaningful analytics ready structures.
Finally, their scalability allows for real-time operations on massive datasets, making them ideal for applications ranging from urban planning to disaster management and environmental monitoring. This combination of efficiency, intelligence, and scalability positions embeddings as a foundational shift in our work with geospatial data.
Unprecedented Scalability
As geospatial data grows exponentially, from UAVs, LiDAR, satellite imagery, IoT sensors, and more, embeddings compress complex geospatial data into compact formats, enabling large-scale storage and processing without losing valuable insights, essential for industries like disaster response and autonomous navigation.
Seamless Interoperability
Embeddings unify diverse data formats into a shared structure, allowing, for example, a satellite image to align perfectly with a BIM model or a textual description. This removes manual integration and simplifies workflows, enabling faster, richer analyses across various geospatial data sources.
Hyper-Searchable Environments
Embeddings enable the creation of hyper-searchable geospatial environments by seamlessly integrating geospatial data with other contextual information. For instance, a natural language query like “a river surrounded by dense vegetation” can directly inform a search.. By integrating datasets like climate and geospatial information, embeddings transform insight generation and decision-making into a cohesive and searchable framework, redefining how we interact with spatial data.
Semantic Understanding
Traditional GIS systems treat features as data points with attributes. Embeddings, however, enable semantic relationships, allowing AI models to infer that a “park” near a “school” impacts traffic patterns differently than a “shopping mall” near a “train station.” This leap from spatial to contextual reasoning is a game-changer.
Real-Time Analytics
Embeddings allow for rapid similarity searches, clustering, and nearest neighbour analyses. Imagine being able to instantly compare urban areas worldwide for disaster preparedness based on terrain and infrastructure embeddings or query a global map for all roads with “urban alley-like conditions” for autonomous vehicle training. Unified embedding models can detect anomalies like illegal deforestation or monitor large infrastructure constructions in real-time.
AI-Powered Automation
Embedding geospatial data eliminates the need for extensive preprocessing and manual effort to clean and align datasets for machine learning. By creating a format that AI systems can directly process, embeddings pave the way for fully autonomous systems. This enables smart cities to adapt dynamically to real-time factors like traffic, weather, and human activity, while automated monitoring pipelines detect and predict changes without time-consuming preparation. This transformation significantly streamlines workflows, allowing AI to fully unlock the potential of geospatial intelligence.
Applications That Will Define the Future
Embeddings are revolutionising how geospatial data is processed, analysed, and applied, enabling new ways to interact with spatial information. Below are key areas where they are set to redefine the geospatial landscape:
Digital Twins: From Static Models to Predictive Systems
Digital twins, widely used in construction, energy, and logistics, are evolving from static replicas into dynamic systems powered by embeddings. By integrating real-time data from sensors, IoT devices, and satellites, embedding-driven digital twins can simulate scenarios like energy consumption, traffic flow, or structural integrity under extreme weather. These systems become predictive tools, offering actionable insights beyond simple monitoring.
Change Monitoring at Scale: Precision in a Dynamic World
Monitoring changes across vast datasets, such as satellite imagery or daily LiDAR scans, is resource-intensive. Embeddings simplify this process by enabling precise, scalable comparisons. They allow rapid detection of deforestation, urban development, or infrastructure damage, capturing both large-scale and micro-level changes. Paired with machine learning, embeddings help prioritise critical changes, streamlining resource allocation.
Generative Geospatial Design: Building Smarter, Faster, and Better
Generative AI powered by embeddings can transform design processes by creating optimised layouts or scenarios with remarkable speed. Urban planners can input constraints like population density or environmental impact, and the model can propose ideal city layouts or transport networks. Similarly, ecological simulations can predict the effects of infrastructure projects, enabling sustainable decision-making. This approach allows for iterative planning and rapid evaluation of multiple scenarios.
Searchable 3D Reality Models: Query Insights in Large-Scale Spatial Data
Massive 3D models of real-world environments are becoming standard, but their usability is often limited by manual inspection. Embeddings make these models fully searchable and actionable. For instance, an engineer could locate specific bridges in a floodplain, or a manager could identify traffic hotspots overlapping with delivery routes.
When combined with large language models, embeddings enable natural language queries. A construction manager might ask, “Which areas are behind schedule, and how does this affect the budget?” The system could integrate 3D scans, timelines, and financial data to deliver actionable insights. This fusion of embeddings and language models creates intuitive, conversational interactions, streamlining decision-making in complex geospatial environments.
What Needs to Happen Next?
The potential of embeddings in the geospatial industry is clear, but fully realising their value requires overcoming key challenges:
1. Standardisation
Developing industry-wide standards for geospatial embeddings is essential to avoid fragmented systems and ensure interoperability—the very strength of embeddings. Without this alignment, their transformative potential will be limited.
2. Rethinking Data Pipelines
Traditional workflows are not designed for embedding-rich, AI-driven platforms. Geospatial organisations must invest in infrastructure that supports these advanced processes, enabling more efficient handling of embedding-based workflows.
3. Embracing the Human Factor
For embeddings to succeed, geospatial professionals need to adopt AI and machine learning as everyday tools. This involves more than upskilling; it requires fostering a mindset that views AI as a collaborator in solving complex spatial problems. The industry must also look beyond its traditional boundaries, learning from innovators like Niantic and NVIDIA,, who are embedding geospatial intelligence into gaming, simulation, global scale digital twins and advanced AI applications.
4. Ethical Considerations
Embedding-driven approaches must prioritise privacy, fairness, and accountability. Establishing robust validation mechanisms is critical, especially with generative AI, to ensure accuracy and maintain trust.
By addressing these challenges, the geospatial industry can fully embrace the opportunities embeddings offer, staying competitive and forward-thinking in an evolving technological landscape.
Why This Matters for Sensat
Sensat is at the forefront of driving transformative change in geospatial technology. As a geospatial cloud-based SaaS platform, we blend advanced 3D visualisation with seamless collaboration, providing project teams with unparalleled access to actionable data and insights. Our foundation as a geospatial data company, built on decades of expertise in data capture, processing, and AI integration, positions us uniquely as both a leader in visualisation and a pioneer in advanced geospatial intelligence.
Embeddings are the cornerstone of the next generation of geospatial innovation, and at Sensat, we envision a future where geospatial queries are instant, precise, and scalable. Imagine stakeholders asking, “Where is the best place to build?” and receiving answers that unify spatial, contextual, and predictive data. Embeddings enable the detection of meaningful changes in complex environments with pinpoint accuracy and the optimisation of networks through multi-modal data integration. This evolution transforms workflows from manual and reactive to predictive, automated, and deeply insightful.
For us at Sensat, this is more than a technological shift, it is a reimagining of how we interact with the world. Embedding-driven geospatial intelligence fosters a deeper understanding of spatial relationships, contextual interdependencies, and temporal dynamics, bridging the gap between raw data and actionable decisions.
Final Thoughts
Just as search engines unlocked the power of vast, unstructured web data and transformed how we navigate information, embeddings promise to revolutionise how we interact with geospatial data. The rise of embeddings marks a turning point for the geospatial industry, offering a pathway to break free from traditional constraints and unlock new possibilities. Beyond their technical sophistication, embeddings represent a shift in perspective, one that prioritises connections, context, and integration over isolated data stores. They enable us to transform geospatial intelligence from a static asset into a dynamic, adaptive resource capable of addressing real-world challenges at scale.
For the geospatial industry, this is an opportunity to rethink its role in a rapidly evolving world. By embracing embeddings, we move closer to an era where insights are no longer bound by data silos or manual processes, but instead emerge naturally from interconnected systems. This is a moment to lead with innovation, collaborate across industries, and ensure that technology serves not only efficiency but also sustainability and equity.
At Sensat, we are committed to turning this vision into reality, building tools that make geospatial intelligence more intuitive, actionable, and impactful. The future of geospatial data is not just about what we can map but what we can achieve with the understanding, and embeddings are the key to that future. Watch this space.
Want to be kept updated about Sensat Geospatial Data innovations? Sign up here to receive the latest news.